Iterative learning for efficient additive mass production
Additive manufacturing (AM), or 3D printing, has long been celebrated for its ability to create complex, customised products on demand. However, its application in mass production has been hindered by reliability issues. Our latest research with the University of Cambridge introduces an iterative learning framework that harnesses AI to enhance the efficiency and precision of AM processes.
The developed system utilises a baseline AI model trained on a diverse range of 3D-printed parts, which then specialises in a specific part by continuously learning and adapting throughout the production process. This approach, known as Iterative Learning (IL), allows the AI to refine its understanding and control of the manufacturing process with each successive build cycle.
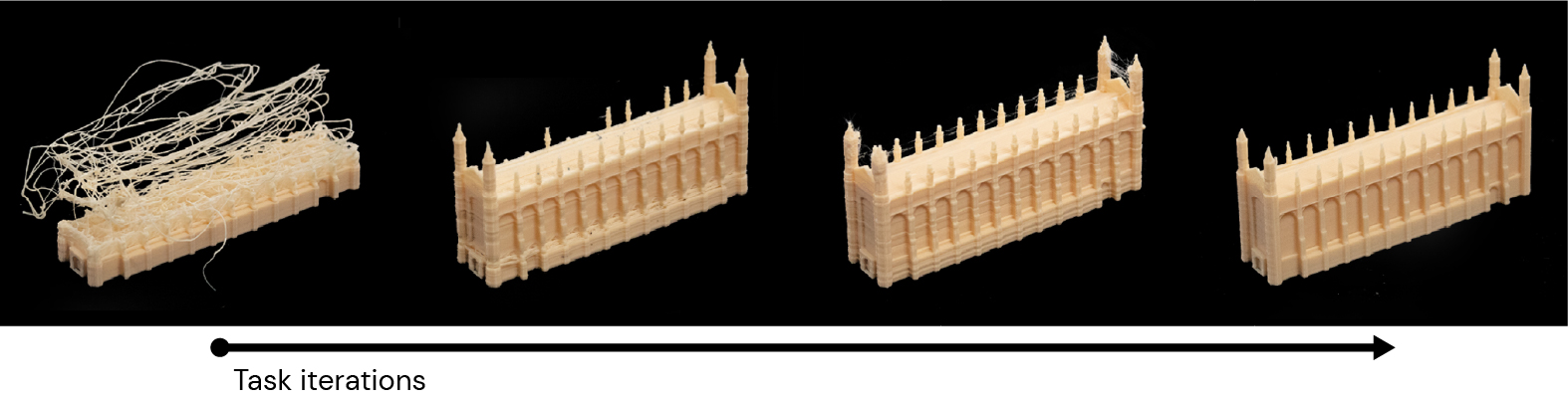
The reliability challenge in additive manufacturing
Material extrusion is one of the most widely used AM techniques. It works by extruding material through a nozzle, layer by layer, to build a part. While this method is versatile and cost-effective, it often struggles with consistency, particularly in high-volume production. Variability in material quality, changes in environmental conditions, and differences between machines can introduce errors, leading to wasted materials and time.
Traditionally, addressing these errors has relied on manual intervention, with skilled operators adjusting parameters through trial and error. However, this approach is not sustainable for large-scale production, where real-time, automatic adjustments are necessary to maintain quality and efficiency, particularly across a fleet of machines operating around the clock, 365 days a year.
Our approach: Iterative Learning
Our research introduces an innovative method called Iterative Learning (IL) to the mass manufacturing context. This integrates deep learning with real-time control systems. The heart of this approach is a foundation model—a type of deep learning model pre-trained on a broad range of tasks. This model serves as the starting point for our IL framework, which continually improves its performance by learning from each build cycle.
The process works as follows:
- Vision-Based Monitoring
We equipped the AM system with a vision-based control system that uses a camera to monitor the material extrusion process in real-time. This setup captures detailed images of the extrusion process, providing a rich data source for error detection.
- Foundation Model
The foundation model, pre-trained on a wide range of 3D printed parts, is initially used to control the printing process. It begins with a general understanding of how to manage the extrusion but lacks the specific knowledge needed for optimal performance in the mass production of the same part.
- Active Learning and Uncertainty Awareness
As the printing process continues, the system employs active learning to refine its model. It actively seeks out new data by focusing on areas where it is most uncertain. By recognizing its own uncertainties, the system can request additional information or make more conservative adjustments, reducing the likelihood of introducing new errors.
- Iterative Learning Process
After each build cycle, the system analyses the results, identifying any errors and the conditions that led to them. This information is fed back into the model, which is then fine-tuned using the new data. The process repeats, with the model becoming progressively more accurate and reliable with each iteration.
- Error Correction
The system doesn't just detect errors; it actively corrects them. By integrating a probabilistic control algorithm inspired by Monte Carlo localization (MCL), the system can make informed corrections in real-time, ensuring that the material flow rate and other critical parameters stay within optimal ranges.
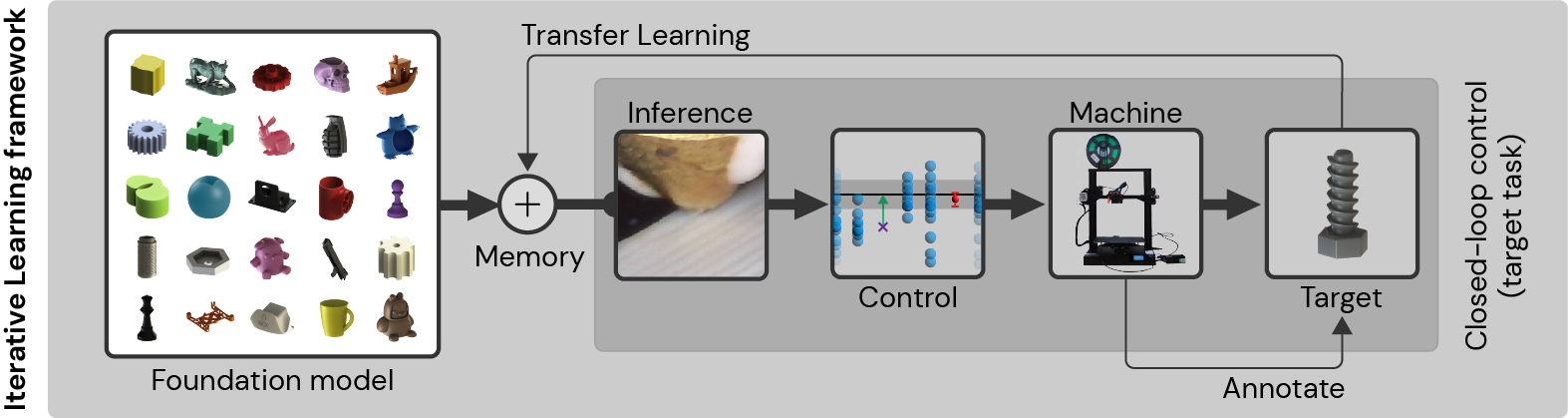
Why Iterative Learning matters
The IL framework represents a significant advancement in AM technology. Traditional machine learning models often struggle when faced with new tasks or conditions. In contrast, our IL framework is designed for this challenge, continuously improving its accuracy and adaptability with each new iteration of a task. For manufacturers, this means fewer failed prints, less wasted material, and more reliable production processes—key factors for scaling up AM in demanding industries like aerospace, medical devices, and construction.
Moreover, the incorporation of uncertainty awareness allows the system to operate more cautiously when necessary, avoiding over-corrections that could compromise the quality of the final product. This approach to error correction ensures that interventions are not only timely but also appropriate for the specific situation.
The future of mass production in AM
This research provides an example for how AI can transform manufacturing, where intelligent systems can adapt and improve continuously. The iterative learning framework we've developed is not just a tool for enhancing AM—it's a model that can be applied across manufacturing technologies. By combining real-time data collection, active learning, and iterative refinement, we can achieve a level of precision and reliability in mass production that was previously unattainable.
As we continue to refine this technology, its potential applications extend beyond AM to other areas of manufacturing that require high precision and adaptability. The iterative learning approach offers a powerful new way to optimise production processes, making it possible to meet the demands of large-scale, high-quality manufacturing in a wide range of industries.
Stay tuned as we continue to explore the possibilities of this groundbreaking technology and push the boundaries of what's possible in manufacturing.
Further Reading
Read the paper
Authors
Christos Margadji
Douglas A. J. Brion
Sebastian W. Pattinson